Predictive sales analytics: The smart seller's key to boosting business growth
- Last Updated : December 16, 2024
- 32 Views
- 9 Min Read
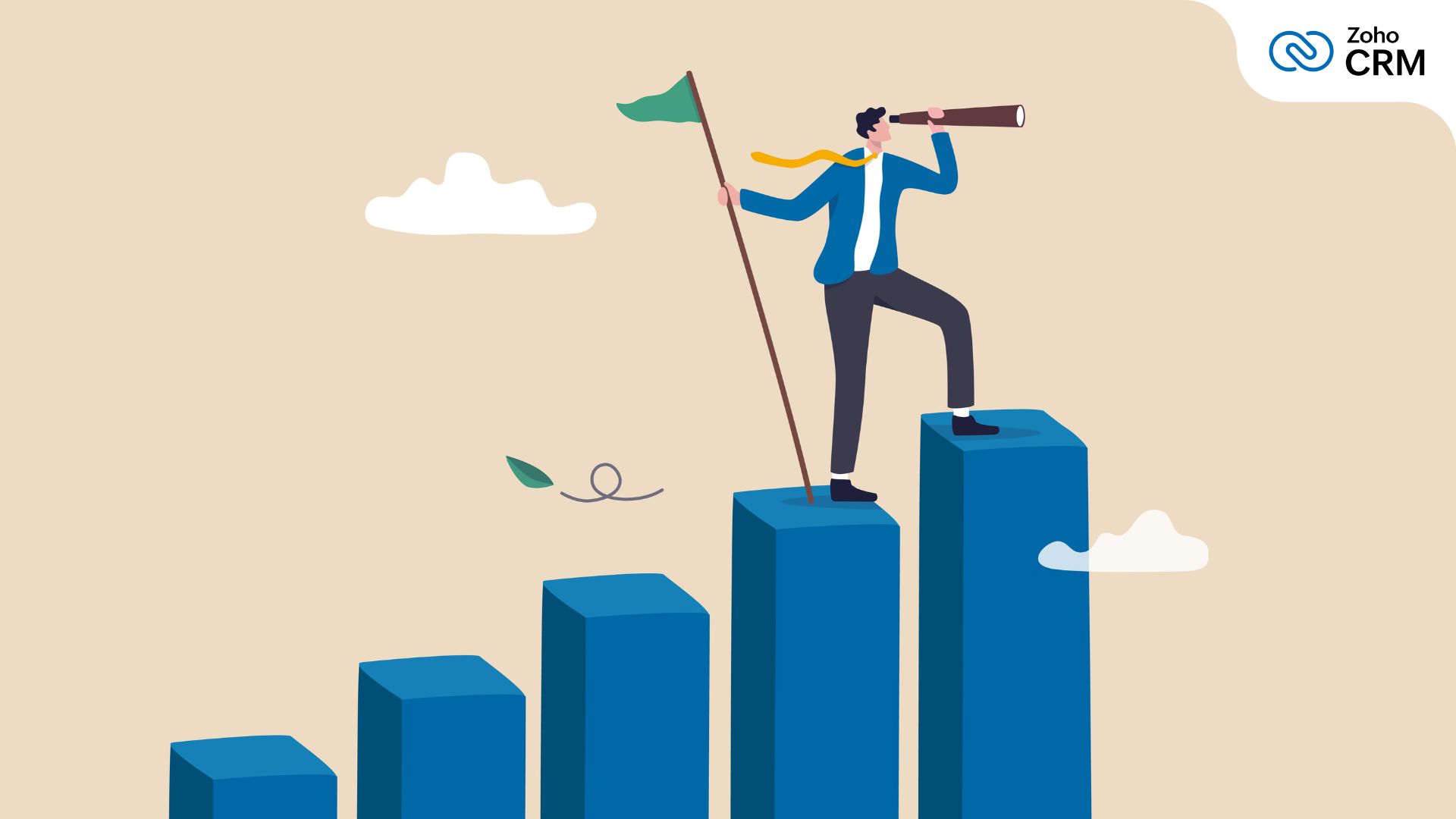
Imagine you're planning a weekend vacation for your team. You'd probably look up the weather forecast for your destination before planning activities for your team—or if you're driving through some of the busiest junctions in the city, you'd use a GPS navigation system to find out where traffic is building up so you can use alternate routes, right? While these two use cases have straightforward solutions, predicting and charting business growth is hardly straightforward.
Various external factors, such as market demand, customer preferences, and economic conditions, as well as internal factors, like resource availability, seller workload, operating costs, and top management buy-in, all profoundly impact your business. It's impossible to predict how each of these factors together and individually can affect your business. However, sellers can leverage predictive sales analytics to foresee changes and drive their businesses forward despite these challenges.
In this article, we'll cover the basics of predictive sales analytics and how it helps forecast business growth, as well as explore a few real-life applications where it can help drive positive change and growth.
What is predictive sales analytics?
Simply put, predictive sales analytics is the ability of sellers to predict future sales—which products and how many units to sell across regions, business units, or sales teams. Predictive sales analytics is a tectonic shift towards proactive business management instead of the traditional reactive management where businesses play catch up with trends and market changes. Most importantly, predictive sales analytics equips leaders with a better viewing lens to predict the future accurately.
At its core, predictive sales analytics is an analytical technique—powered by predictive algorithms—to create forecasts that predict future sales using historical data on sales figures, revenue, resource availability, territorial agent distribution, product diversity, and more.
Any predictive sales analytics that's performed manually is error-prone due to human biases and fallibility, data inaccuracies, or the use of poor predictive modeling techniques. AI-led predictive sales analytics has clear advantages due to its speed, accuracy, and bias-free results, as well as its ability to analyze enormous data volumes to produce reliable forecasts.
The impact of predictive sales analytics on your business
Sales forecasting is important to the business in two critical areas: It serves as a spotlight to identify potential growth areas and acts as an early warning system for critical risks and problems.
Sales forecasting can guide strategic plans, plan resources, predict market demand, align inventory to dynamic customer demands, and thus minimize operational costs and maximize profits.
By tracking historical records, predictive sales analytics can identify potential pitfalls before they build momentum. Upon comparing patterns and trends in current data, it can catch deviations early on, alert users, and buy the organization much-needed "buffer time" to resolve potential bottlenecks.
Driving growth through predictive sales analytics
Predictive sales analytics encompasses all aspects of sales management, starting with sales planning and including revenue forecasting, anticipating customer behavior, predicting market demand, designing better marketing campaigns, and forewarning of pending risks. Now, let's explore some practical use cases for how predictive sales analytics can help drive sales and craft better sales strategies and tactics.
Lead scoring
Not all leads that come into your CRM convert into customers, so unless you have a ton of resources and time to burn through, you'll want your sales efforts to be focused on promising leads so you can make the right bets. Predictive lead scoring helps businesses stay agile and focus their efforts on true prospects and not on leads who are casually browsing through your catalog with no purchase intent.
Predictive lead scoring is achieved through two means: Either businesses put in the time and effort to manually configure the scores for expected actions, events, and behaviors that customers normally exhibit before making a purchase, or they leave it to AI algorithms to parse customer information automatically and analyze trends in past purchase histories to create a scoring mechanism that awards appropriate scores to leads. While the manual process can be taxing, biased, and error-prone, AI-led scoring is reliable and saves countless man-hours every day. An AI-based scoring system can also watch out for signals in related data that's obtained by integrating your CRM with other data sources, such as your help desk, marketing automation platform, website visitor information tracking application, and more. This ensures that lead scoring is comprehensive and takes into account every single interaction your leads have with your brand.
Brigade Plus, an interior development and real estate company, uses Zoho CRM's predictive lead scoring rules to prioritize leads based on customers' predicted level of interest. Along with some of other automation features, such as workflows and automated suggestions on the best time to contact leads, this saves the company up to 18 hours every week. Read the full story here.
Deal propensity
Having a bucket load of qualified leads doesn't guarantee purchases. Most of those leads may not even get to the purchase stage. Stats say that the average conversion rate for ecommerce is 4%, which means nearly 95% of the leads who interact with your brand will likely never make a purchase.
So unless you have a system that enables you to identify which leads are likely to convert, you'll likely approach all your leads with generic sales strategies. Without any personal touch, your leads might not feel any connection with your brand.
Almost like a crystal ball, predicting deal propensity shows your business which deals have a greater probability of converting based on typical buying behavior and enables you to allocate resources toward deals that matter to your business. This also ensures that promising leads get the full-fledged, personal attention of the sales team, thereby improving your chances of success.
Besides predicting the likelihood of deal conversion, it's also possible to get reasonably accurate predictions for the number of days it will take to convert deals. Better yet, these deals can also be grouped by the time required (as predicted) to convert them, making it all the more convenient for your sales team to prioritize them.
Cross-selling and upselling
Cross-selling and upselling to existing customers are cost-effective ways to boost your organization's revenue quickly. By providing customers with the right solutions at the right time, these methods can also help increase customer satisfaction.
Predictive analytics assesses historical buying behavior to understand which customers buy from your brand and when, and then uses this information to accurately predict when customers are ripe for cross-selling or upselling initiatives—enabling businesses to personalize offers and provide lucrative discounts. Predictive analytics can also be extended to first-time customers using knowledge obtained from existing customers.
One simple application of predictive analytics involves predicting that customers who buy battery-operated devices, for example, are likely to purchase spare batteries after a few weeks. A slightly more complex use case is using predictive analytics to foresee that customers who purchase a CRM solution may need an analytics application a year after implementation.
Customer profiling and clustering
Enterprises can benefit from clustering customers into groups for better targeting and engagement. The objective of predictive profiling is not to isolate the behaviors of a single customer, but rather to understand identical behaviors among groups of customers with similar backgrounds, such as industry, geography, company size, revenue, time taken to close deals, responsiveness, interests, and more. This helps businesses identify the next best actions and prioritize resources for time and improved outcomes.
Unsupervised AI-led customer profiling and clustering can be used to place customers in the right pipelines and fuel the right sales efforts to boost sales. Businesses can also use it to identify the right target groups for various product categories. The illustration below highlights how customers from various age groups perceive adventure sports among various service packages offered by a hotel, as measured by their happiness index.
Targeted discounts
Businesses can resort to discounts as a means of closing long-pending deals, boosting revenue quickly, undercutting competition in established markets, or satisfying customers who are price sensitive. Particularly in the ecommerce sector, where competition is heavy and customer loyalty is fragile, targeted discounts help stay one step ahead of the competition and help facilitate buying decisions.
Businesses can use predictive analytical algorithms to analyze customer behavior, demographics, and buying history to forecast future buying patterns, and then leverage this information to create targeted discounts for the right groups of customers at the right times—thereby increasing the efficacy of marketing campaigns and improving sales margins without compromising on profit margins.
Customer churn prediction
Organizations can also use predictive analytics to predict when customers are likely to leave a brand. By watching out for signals such as changes in customers' interests, waning or vanishing interest in the product or service, poor product usage levels, low trust, or negative interactions with the brand, brands can predict churn and boil it down to a numerical score. The higher the churn prediction score, the greater the chance is that the customer will leave the brand.
Some CRM applications, such as Zoho CRM, provide granular visibility into the set of behaviors and the individual scores that contributed to the overall churn prediction score. This makes it easier for the sellers to approach customers with personalized messages that can help change their perception and turn deals around.
Revenue forecasting
Revenue forecasting isn't just about using historical sales data to predict future sales or revenue; it's the fulcrum on which businesses can plan their growth trajectory, expansion, and market penetration. Revenue forecasting gives business leaders the foresight to align org-level objectives throughout the organization and ensure that every team within it contributes effectively.
Revenue forecasting takes a top-down approach, with senior management charting the overall strategies and plans and each team or business subset chipping in with their own contributions and ideas. For small and medium enterprises, revenue forecasting helps plan resource allocation and marketing strategies, finalize budgets, and manage cash flow more effectively. For large enterprises, revenue forecasting is useful for shaping the company's future and chartering new routes for growth.
It's difficult to overstate the importance of revenue forecasting. Per Clari's 2024 Revenue Leak Report, nearly 61% of sales teams missed their revenue targets for 2023, and 71% of the teams surveyed admitted that their forecasts were erroneous. Accurately predicting revenue and following it up with strategies and tactics can help organizations attain revenue goals and arrest revenue leaks.
Sales planning
While revenue planning tells your company where it should be in a year, sales planning charters the path towards growth and outlines how the company should get there. Sales planning involves simulating and testing strategies and making adjustments to ensure you achieve your targets. For instance, sales managers for each region can break down their overall revenue target for their regions and split the targets among various zones, sub-regions, or salespeople. This helps split the target and focus resources and time into areas with the most potential. Sales managers can also determine how much of the target revenue can come from existing customers by way of recurring sales or upgrades, and how much requires new sales to hit the targets. This helps shape sales and marketing efforts.
Teams can also track target achievement and create alerts to catch deviations from planned targets. AI-led achievement predictions are great at catching deviations well ahead of time so that sales leaders can course-correct or pump in additional resources to get back on their planned trajectories.
Integrating predictive sales analytics into your operations with Zoho CRM
Businesses have always wanted that crystal ball that enables them to look into the future, predict trends, foresee customer behavior, anticipate market changes, and design marketing, sales, and support strategies that drive better business outcomes and leave a trail of happy customers.
Zoho CRM features a robust prediction engine—powered by Zia, its built-in AI assistant—that enables sales teams to foresee and plan all sales activities, including sales planning, offering personalized discounts, predicting churn, and retaining customers. Zia offers the following functionalities:
- Sales prediction: Zia can predict with high accuracy the likelihood of deals converting, which makes it easier to streamline pipelines, plan sales operations efficiently, and distribute resources and time for the right deals.
- Anomaly detection: Get instant notifications when sales activities and revenue figures don't match your plans. Revisit plans and revamp strategies to get back on track and hit those revenue targets without fail.
- Customer profiling and segmentation: Track every word, email, or call from your customers. Compile all that data into a dashboard to understand your customers in depth; create cohorts and segment customers based on criteria specific to your business, and then provide solutions that solve their problems.
- Customer churn prediction: Proactively predict churn by accounting for churn-inducing factors among customers' behaviors. Counter these with churn-preventing factors that Zia automatically generates and create long and meaningful relationships with your customers.
- Prediction builder: Configure predictions for standard and custom modules and define rules for how those predictions should work. Guide and tune Zia to focus on data that matters to your business and create predictions that drive it forward.